
Medicasci: Deep Learning Semantic Data Lake for Clinical Decision Support
Sepsis is the leading cause of in-hospital mortality, where one in three inpatient deaths are due to sepsis. Incidence of sepsis has been increasing with 1.7 million sepsis cases and 270,000 deaths per year. More than 5 million patients are admitted annually to United States ICUs with average mortality rate reported ranging from 8-19%, or about 500,000 deaths annually. Early identification of deterioration has been shown to reduce the need for patient transfer to higher care units, reduce lengths of stay, and improve survival rates.
Medicasci is a cloud-based deep learning system for context-sensitive clinical decision support in monitoring and predicting the deterioration of patient health and progression of sepsis risk factors in real-time to improve outcomes and optimize the management of care across the hospital population. To support the clinical care team, Medicasci provides team members with:
- AI models for predicting septic conditions over multiple time frames
- Early warning scores for deteriorating health conditions
- Evidence-based clinical practice guidelines to improve patient care
- Visualization of patient health status trends
Medicasci was funded in part by National Institute of General Medical Sciences grant 1R44GM143996.
GeneTegra® Enterprise Knowledge Management
GeneTegra Enterprise Knowledge Management combines our innovations in semantic data integration, knowledge modeling, high-throughput computing, and artificial intelligence to solve problems in extracting actionable intelligence from diverse data sources regardless of data formats, semantic and syntactic structures, and location.

GeneTegra Semantic Integration is a novel semantic information integration system that reduces the time needed to locate and browse biomedical data from disparate sources, ensure its quality, assemble it into meaningful datasets, and analyze it using complex machine learning tools, leading to a significant increase in research output. GeneTegra leverages our ASMOV ontology alignment component and provides automated semantic modeling of heterogeneous data sources. This is accomplished without having to create a physical data warehouse. GeneTegra improves turnaround time and reduces the time spent on manual data management and data abstraction processes. With its on-demand integration and sharing capabilities, GeneTegra enhances team-based research and reduces the existence of data silos.
GeneTegra® Key Features
![]() |
Easily view, create and share data among researchers and scientists throughout an institution | ![]() |
Allow access to multiple databases as a single harmonized view |
![]() |
Re-purpose data by customizing the views to support new research endeavors | ![]() |
Explore and query organizational data more efficiently |
![]() |
Ensure data freshness by distributing the queries among live data stores | ![]() |
Utilize consistent terminology to access unfamiliar data |
![]() |
Resolve inconsistencies between disparate sources through the application of rules | ![]() |
Combine personal research data with institutional resources |
GeneTegra® Applications
GeneTegra has been designed and deployed to support clinical and research applications in the life sciences.

ASMOV, which stands for Automated Semantic Mapping of Ontologies with Verification, is an automatic ontology alignment tool which produces class-to-class, property-to-property, individual-to-individual mappings. Although it was originally designed to facilitate the integration of heterogeneous systems, using their data source ontologies, it can be used to align ontologies from any domain.
Due to its iterative nature, ASMOV is able to analyze the intermediate alignments produced and eliminate incoherencies whenever found. This feature positioned ASMOV as one of the top three alignment tool at OAEI (three years in row) and as the most coherent alignment tool available.
User Feedback
Although ASMOV is an automated process, it does not ignore the user interaction aspect that may be required to obtain perfect alignments. As a result, the ASMOV User Interface include a component that allows the user to review the alignments generated and address the errors that may exist. Since a single change may render a alignment incoherent, ASMOV will revalidate the alignment while considering the user inputs as partial alignment.
Background Knowledge
By default, the lexical matcher of ASMOV, which calculates the lexical similarity between entities, uses WordNet. However, other background knowledge may be more appropriate for other domains; for example, UMLS is better suited to alignment medical ontologies. Consequently, ASMOV defines interfaces that enables expert users to implement adapters to other background knowledge.
Logical Reasoning (Semantic Verification)
The Semantic Verification component within ASMOV make it possible to self validate the pre-alignments, which lead to a more accurate alignment. The five types of verifications that are performed are illustrated below:
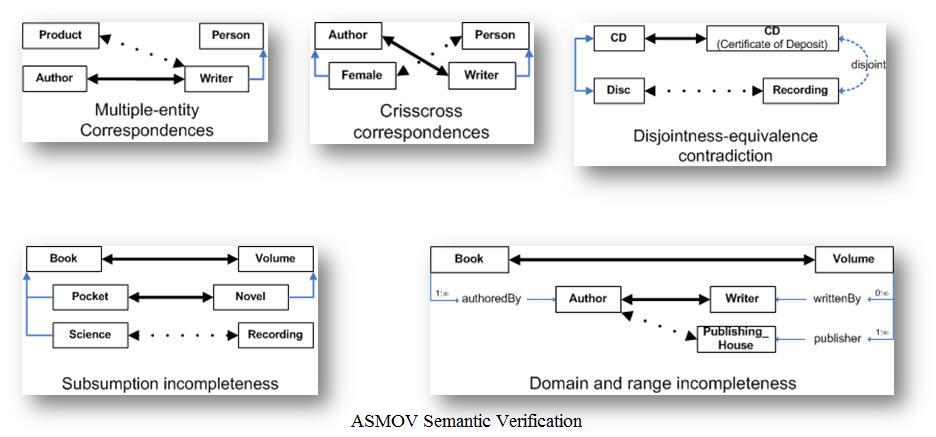